AI is a mirror of our world. It ‘creates’ (though to say that it amalgamates is more accurate) using available data, appearing neutral. But the data – images and language used – is not neutral. We know that data sets contain biases against non-dominant societal groups, namely women and people of colour. ChatGPT, Bard, Midjourney, DALL-E, Gemini are all inherently biassed. Why? Because that’s the reality of the world right now, and of the history leading up to this moment. Numerous causes are contributing to this issue: incomplete and skewed datasets and incorrect labels used for training AI. This matters, because AI is supposed to be helping us to progress - not to reproduce or further embed biases we’re trying to eliminate.
Deepti Velury, Tag’s global chief operating officer, points out that when it comes to AI “if we put garbage in, we get garbage out. This is how any data works, and this is where the fundamental issue of gender bias in AI arises. Because the data is coming from humans who, by nature, hold biases, it’s impossible to expect the output to be anything other than this.” However, this should be seen as a starting and not the end point with Deepti stating that the current state of things “doesn’t mean that we can’t get it right.”
Data bias
For Erin Sarofsky, the founder of motion design studio Sarofsky, AI’s biases are patently clear. They’re a reflection “of all the content that's been created by humans. Anything that's been published and accessible is the dataset it’s using to build from. So it’s reflecting exactly where we are as a species. Ask yourself who has primarily published content over time and what their worldview is or has been, and that’s where AI is at.”
Odile Berthon, owned media manager at Locaria - a multilingual content activation agency - shares Locaria’s findings from continuous testing of LLMs (large language models) like ChatGPT and Bard. “Our testing reveals a clear pattern in LLM output: AI engines, trained on publicly available content, are only as good as the data that feeds them and tend to reproduce and propagate gender bias. In a world where social biases are unfortunately common, we cannot assume that an AI engine would be able to neutralise them. It’s quite the opposite: the higher the volumes created via GenAI, the more such biases are replicated and propagated, often with little human supervision. This may feed a vicious circle, as future models ingest AI-generated content too. So far, technological progress does not directly equal societal progress.”
MullenLowe Group’s global chief information officer Veronica Millan likewise suggests that the historical and cultural baggage AI models are trained on do not produce results for a world we want to see, but merely the one we find ourselves in. “The language and art that the model has been trained on is biassed so that when we ask it to give us examples of a person performing or representing an act, we often get the stereotype. Asking generative AI to give us an image of a ‘chef’ might result in an image of a man wearing a white coat in a professional kitchen, but a request to create an image of ‘a person cooking’ might be a woman in the kitchen by herself, possibly wearing an apron.”
When Odile asked ChatGPT to describe the day of a CEO and of a nurse, in French, the results didn’t surprise her. “Have a guess which one was described as the male role,” Odile challenges. “Another example: in Japanese emails, my female colleague is addressed with the neutral suffix 様 (sama), which Google automatically translates as ‘Mr’,” she adds. Based on this we can see the assumptions that AI makes - power is coded masculine and the neutral subject is a male one.
AI doesn’t do much better when it does attempt to go against the grain and create beyond the stereotypes that the data influences it to produce. “Just last week, Google ended up in a kerfuffle because their generative AI model Gemini was able to create images that were not ‘stereotypes’ – but resulted in images of people in situations that were historically inaccurate or simply nonsense. Gemini portrayed the United States founders as non-white (racially diverse) and similar results for other periods in time. I’m sure that Google was trying to make sure that Gemini fixed some of the bias that existed in history (for example, men and men of European descent as founders) but they overshot entirely and created falsehoods,” says Veronica. Worryingly, these glitches and hallucinations are not uncommon for AI and many have reported the models presenting falsehoods like fact and insisting on their truthfulness when questioned, which only further highlights how much of a work in progress the models still are.
More work to be done
The infancy of the technology is something Deepti notes too, emphasising just how nascent it really is. “It was only in November 2022 that Sam Altman first launched ChatGPT, therefore we can’t immediately expect the finished article; and this is where humans come in. It is our job to keep evolving, finding the biases and correcting or removing that data with the goal of eventually creating an objective technology free of human and culturally-led bias. This is why human roles become more and more important, taking on the mantle of gatekeepers, checking the output of AI to iron out inconsistencies rather than becoming dependent on a technology which has not yet met its full potential.
While it's humanity’s history that’s responsible for the bias in AI today, the relative nascency of the technology means that there’s time - and an imperative - to influence the technology’s future developments. Erin points out that there is “good news” and that “AI can be challenged to think more inclusively.” For example, “when asking AI for top scientists in a specific field, go further by requesting consideration for women and people of colour (POC).” This individualist approach asks us to continue being aware of our own biases and to employ specificity and intention when interacting with AI.
Yet this only reaches so far. Erin cations: “It's crucial to understand that your individual interactions with AI don't directly influence its future responses to others. Challenging AI to think diversely in one instance doesn't guarantee ongoing change. To truly impact AI, contribute diverse content to platforms like Wikipedia. For women and POC to gain visibility, it's essential they actively engage in telling their own stories online and seize opportunities to share their perspectives publicly. AI can then incorporate their thought leadership into its interactions with users.”
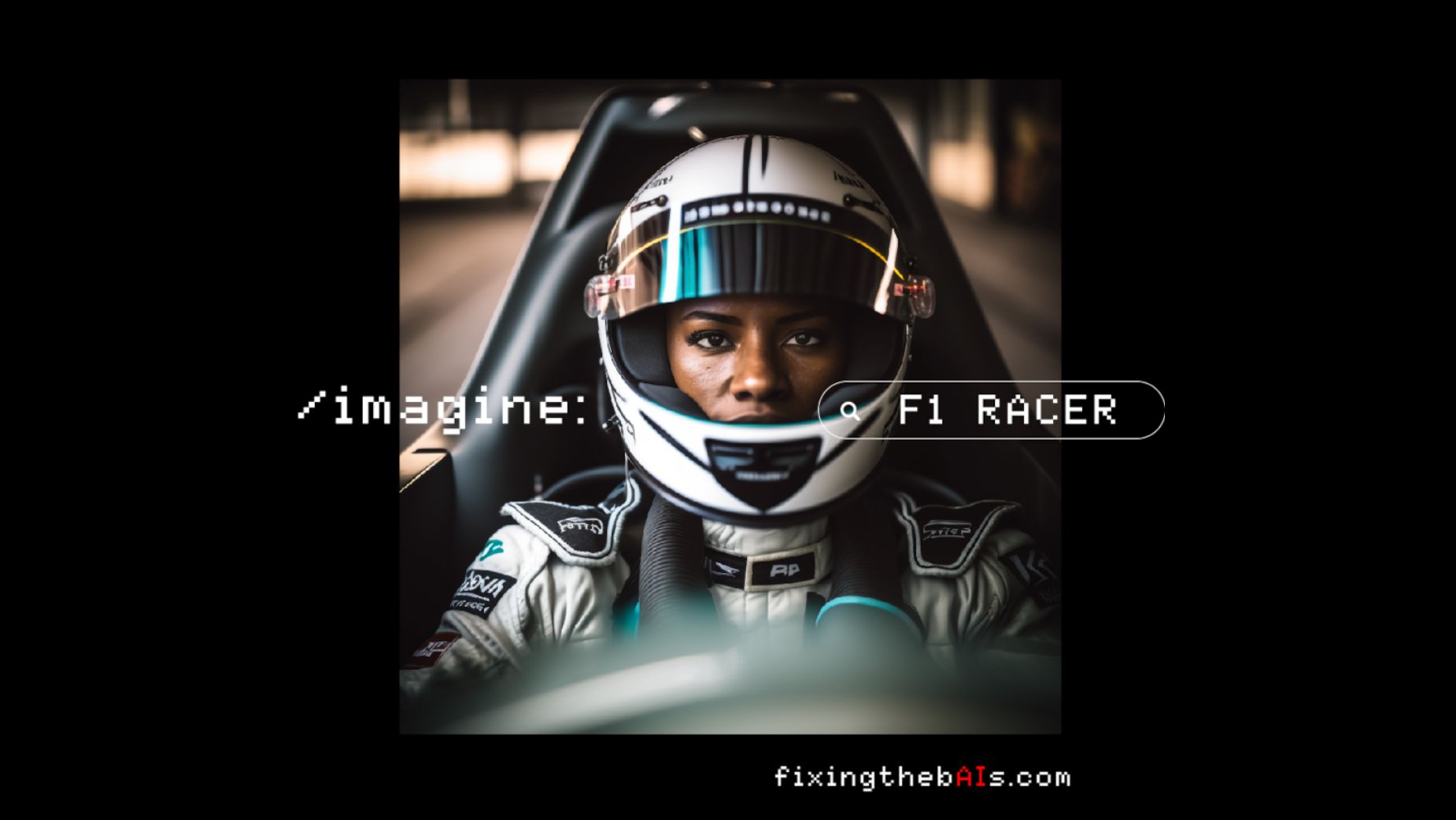
MullenLowe MENA's 'Fixing the bAIs' project.
The answer to improving the technology isn’t more technology for Veronica, and she firmly believes that we “can't automate our way out of bias.” The work that needs to be done has to happen on a societal level and it requires nuance and time. “We, as society and individuals, need to do the work to break the stereotypes that surround us. It’s a start to speak about defaulting to images of women in power, women leading companies, women in positions that are normally left to men, but we also need to do the same for men – to increase the images of men caring for their families, taking care of their homes, and other stereotypical women’s work. And we need to consider how we improve the language we use around genders, to be inclusive, but also to help train these models to consider the nuances and the context of gender norms of the past and the gender norms we are striving and aiming to progress to,” Veronica adds.
Odile likewise stresses the importance of human intelligence and involvement when dealing with anything AI related - it is not to be relied on without being verified, especially for the language side of the industry. “The language industry cannot function without human involvement: only human intelligence can detect bias and correct its linguistic markers. Relying on a pool of specialised, trained language professionals to review machine output is more essential than ever during this AI revolution,” she adds.
Tackling the challenges
Still, AI is being utilised by the industry already and many more companies are in the testing phase to see how it can best serve them and their clients. At Tag, Deepti tells us that AI applications are geared towards clients who are looking for faster, real-time assets. “This will manifest through evolving our production ecosystem with AI at the heart, to improve our clients’ experience as well as making our own employees' lives easier. There is not one tool that fits across all channels, and there are multiple ways of working, but we are carefully testing and quality checking, using the neuroscience technology in our DI Optimise DE&I tool to ensure we check all system led data and bring out any anomalies,” she shares.
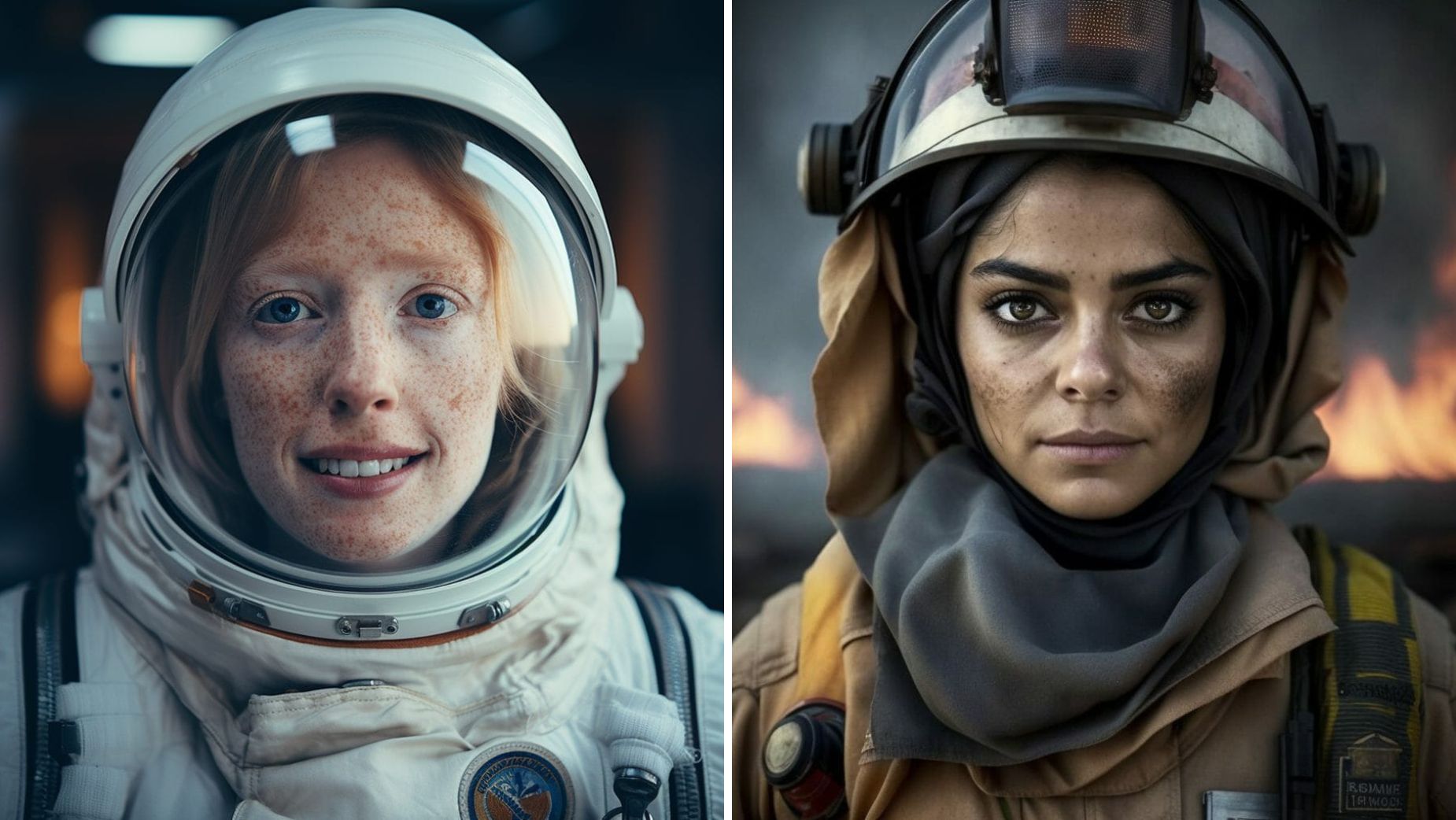
MullenLowe MENA's 'Fixing the bAIs' project.
MullenLowe MENA has been tackling the issue of bias in AI head on. Veronica says: “Our MullenLowe MENA office understood this concern early on and created the campaign “Fixing the bAIs” by building an image bank of women represented in different types of job roles and industries in order to increase the chances of breaking these stereotypes. You can find this database on a
website we built for this and in a
Discord channel, so anyone can continue to add to it.”
It’s positive to see that the industry is aware of the inherent issues embedded within AI and that active steps are already being taken to ensure that gender bias isn’t entrenched further. The progress, perhaps unsurprisingly, is slow and, as always, there is still more work to do. Deepti’s outlook for the future and possibility of a bias-free AI is cautious yet ultimately positive. “The weight still falls on the humans, working in tandem with the data so that process, people and technology form the triad, allowing us to create assets faster, with more accuracy and greater personalisation than ever before.”
While we may think that technological advancements today happen fast, like the increased sophistication of LLMs over the last two years, it’s a different story when it comes to the data that’s needed. Odile notes that “societies take time to change, and data may change at an even more snail-like pace. The battle against sexism will of course continue, with more awareness raised about LLMs and AI.”
Erin concludes: “This process is gradual, and no matter how sophisticated the technology becomes, it is still a long game. Real-world shifts, including the inclusion of new voices, are necessary for AI to learn and evolve toward greater diversity.”